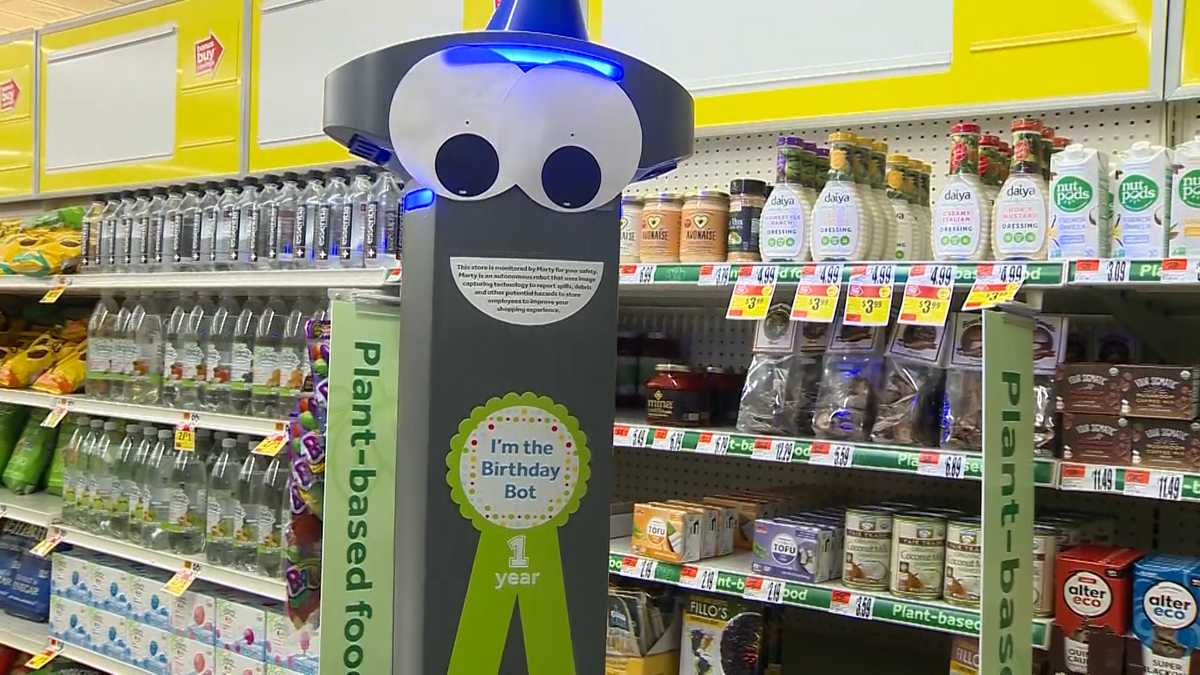
Reinforcement deep learning is a subfield of machine learning that merges reinforcement learning with deep-learning techniques. It considers the problem of a computational agent learning to make decisions through trial and error. Deep reinforcement learning is an upcoming field, but some challenges remain for its deployment. In this article, we'll explore the methods and applications of this type of learning. The next section of this article will focus on the current state and future prospects in robotics.
A goal-directed computational approach
Goal-directed computational approaches to reinforcement deeplearning are based on reinforcement learning. This is a popular paradigm for optimizing Markov decision processes. Reward learning involves agents interacting with their environment to learn how situations can be mapped to actions. The expected cumulative rewards are maximized. This type of optimization requires approximate solution methods, which are often difficult to develop for highly complex Markov decision processes. A recent goal-directed computational approach combines deep convolutional neural networks with Q-learning. Combining both methods results in increased uncertainty, which can be useful in predicting behavior in realtime.
Goal-directed computational approaches teach agents how to interact with stochastic environments and adjust their policy parameters as they observe them. This allows them to determine the best policy for maximizing long-term rewards. Many models can be used to model such agents. To train these algorithms, Reinforcement Learning software is available. These models are not meant to replace human decisionmaking.
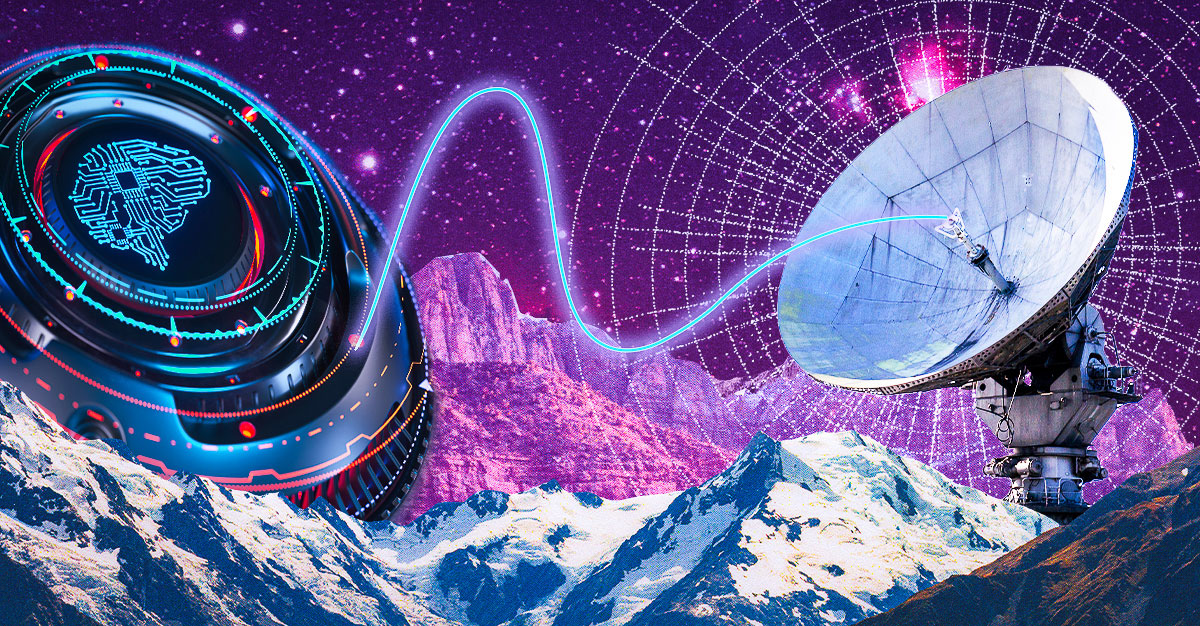
Methods for reinforcement learning
In general, reinforcement deep-learning methods are based on the assumption that agents can be emulated by their environment. Reinforcement learning's objective is to help the agent achieve a predetermined goal. To do so, the agent learns the most rewarding action from a set of data instances. The agent then uses this information in order to improve its prediction. In the next section we will discuss several common methods for reinforcement learning and how they function.
Research community is familiar with several methods of reinforcement learning. The most widely used method in reinforcement learning is policy eration. This method computes a sequence of functions for an activity, which eventually converges on the desired Q*. Other methods can also be used in real-life situations. For more information on reinforcement learning, visit the repo. It's well worth a visit if your interest is in the methods.
Robotics applications
For its potential to simplify manipulative tasks and make robots more efficient at completing them, reinforcement deep-learning in robotics is drawing a lot of attention. This paper describes how reinforcement deep learning can be used in robotics to reduce complexity when grasping tasks. It does this by combining large-scale, distributed optimization with QT-Opt which is a deep variant of Q-Learning. This technique is offline trained and applied to real robots to complete tasks.
Traditional manipulation learning algorithms are complicated to implement, as they require a model of the entire system in advance. The disadvantage of imitation learning is that the strategy learned by imitation is not general enough to handle changing environments. Deep reinforcement learning allows robots to adjust to their environment and make decisions without human supervision. Deep reinforcement learning is an effective option for robot manipulators. The robot manipulation algorithms offer the best options in robotics.
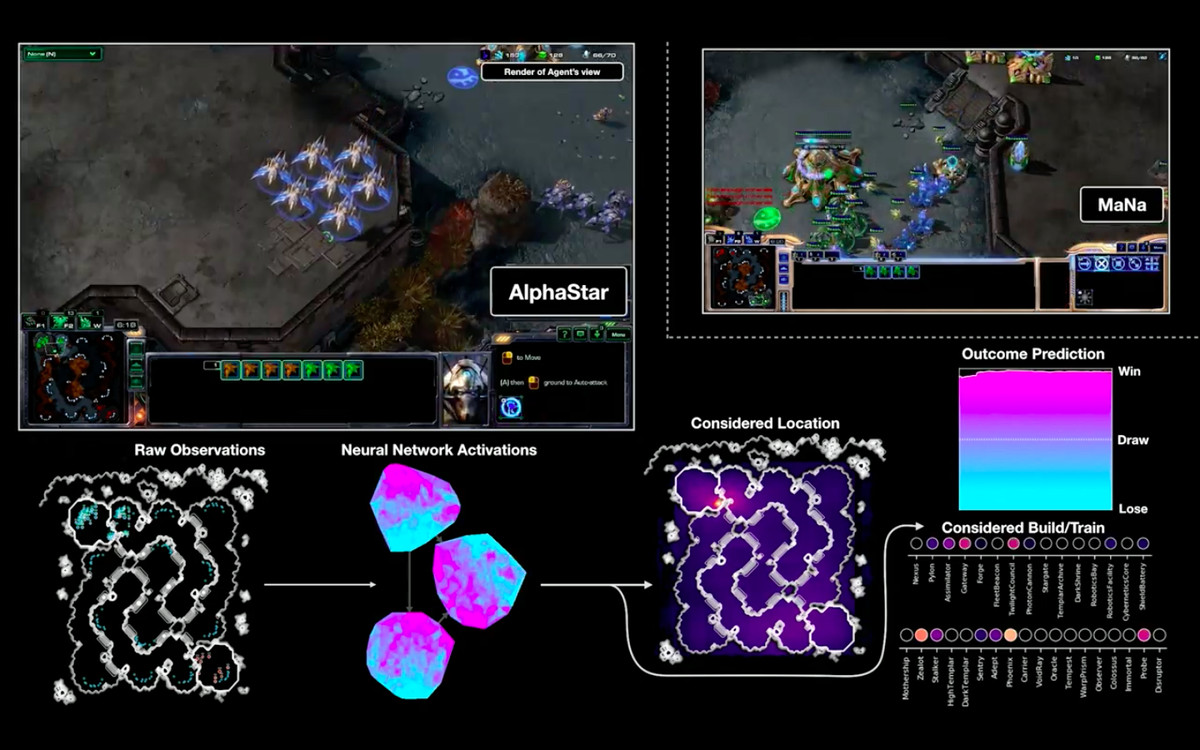
Barriers that prevent deployment
It's not easy to retrain neural networks using new training data. Data scientists need to first determine the environment that they wish to package. The gym, an API for reinforcement-learning, is one common environment that data scientists can use to create packages. The environment is already set up for this task. Data scientists must also be able to integrate other data sources such as images and genomic data.
The Internet of Things generates huge amounts of data. This is because it is a network of billions of connected objects that can communicate with each other and humans. These devices are able to detect human activities, environmental information and geo-information. Due to the large amount of data available, it is essential that we are able to quickly process this data. Fortunately, there are lightweight techniques that can be trained on resources-constrained devices and applications.
FAQ
Which AI technology do you believe will impact your job?
AI will eventually eliminate certain jobs. This includes drivers, taxi drivers as well as cashiers and workers in fast food restaurants.
AI will bring new jobs. This includes business analysts, project managers as well product designers and marketing specialists.
AI will make your current job easier. This includes jobs like accountants, lawyers, doctors, teachers, nurses, and engineers.
AI will make existing jobs more efficient. This includes agents and sales reps, as well customer support representatives and call center agents.
Which industries use AI most frequently?
The automotive industry is one of the earliest adopters AI. BMW AG uses AI as a diagnostic tool for car problems; Ford Motor Company uses AI when developing self-driving cars; General Motors uses AI with its autonomous vehicle fleet.
Other AI industries are banking, insurance and healthcare.
What are some examples AI apps?
AI is used in many fields, including finance and healthcare, manufacturing, transport, energy, education, law enforcement, defense, and government. These are just a few of the many examples.
-
Finance – AI is already helping banks detect fraud. AI can scan millions of transactions every day and flag suspicious activity.
-
Healthcare – AI is used in healthcare to detect cancerous cells and recommend treatment options.
-
Manufacturing - AI is used to increase efficiency in factories and reduce costs.
-
Transportation - Self driving cars have been successfully tested in California. They are now being trialed across the world.
-
Utilities can use AI to monitor electricity usage patterns.
-
Education – AI is being used to educate. Students can use their smartphones to interact with robots.
-
Government – Artificial intelligence is being used within the government to track terrorists and criminals.
-
Law Enforcement - AI is used in police investigations. Search databases that contain thousands of hours worth of CCTV footage can be searched by detectives.
-
Defense – AI can be used both offensively as well as defensively. Artificial intelligence systems can be used to hack enemy computers. In defense, AI systems can be used to defend military bases from cyberattacks.
AI: Good or bad?
AI is seen both positively and negatively. On the positive side, it allows us to do things faster than ever before. It is no longer necessary to spend hours creating programs that do tasks like word processing or spreadsheets. Instead, we just ask our computers to carry out these functions.
On the other side, many fear that AI could eventually replace humans. Many believe that robots could eventually be smarter than their creators. This means they could take over jobs.
What's the future for AI?
The future of artificial intelligence (AI) lies not in building machines that are smarter than us but rather in creating systems that learn from experience and improve themselves over time.
In other words, we need to build machines that learn how to learn.
This would require algorithms that can be used to teach each other via example.
It is also possible to create our own learning algorithms.
You must ensure they can adapt to any situation.
What is the latest AI invention?
Deep Learning is the most recent AI invention. Deep learning is an artificial Intelligence technique that makes use of neural networks (a form of machine learning) in order to perform tasks such speech recognition, image recognition, and natural language process. Google invented it in 2012.
The most recent example of deep learning was when Google used it to create a computer program capable of writing its own code. This was done using a neural network called "Google Brain," which was trained on a massive amount of data from YouTube videos.
This enabled the system to create programs for itself.
IBM announced in 2015 that it had developed a program for creating music. The neural networks also play a role in music creation. These are called "neural network for music" (NN-FM).
Statistics
- According to the company's website, more than 800 financial firms use AlphaSense, including some Fortune 500 corporations. (builtin.com)
- Additionally, keeping in mind the current crisis, the AI is designed in a manner where it reduces the carbon footprint by 20-40%. (analyticsinsight.net)
- In the first half of 2017, the company discovered and banned 300,000 terrorist-linked accounts, 95 percent of which were found by non-human, artificially intelligent machines. (builtin.com)
- The company's AI team trained an image recognition model to 85 percent accuracy using billions of public Instagram photos tagged with hashtags. (builtin.com)
- A 2021 Pew Research survey revealed that 37 percent of respondents who are more concerned than excited about AI had concerns including job loss, privacy, and AI's potential to “surpass human skills.” (builtin.com)
External Links
How To
How to set Cortana's daily briefing up
Cortana is a digital assistant available in Windows 10. It is designed to assist users in finding answers quickly, keeping them informed, and getting things done across their devices.
Setting up a daily briefing will help make your life easier by giving you useful information at any time. The information can include news, weather forecasts or stock prices. Traffic reports and reminders are all acceptable. You have the option to choose which information you wish to receive and how frequently.
Win + I will open Cortana. Scroll down to the bottom until you find the option to disable or enable the daily briefing feature.
If you have the daily briefing feature enabled, here's how it can be customized:
1. Open Cortana.
2. Scroll down to "My Day" section.
3. Click the arrow beside "Customize My Day".
4. Choose which type of information you want to receive each day.
5. Change the frequency of updates.
6. Add or remove items from your shopping list.
7. Save the changes.
8. Close the app